Artificial Intelligence leverages advanced algorithms, machine learning models, and data-driven methodologies to automate processes, extract insights from large datasets, and enhance computational efficiency. Its technical foundation includes deep learning architectures, neural networks, natural language processing (NLP), reinforcement learning, and probabilistic models. These systems rely on high-performance computing, parallel processing, and specialised hardware such as GPUs, TPUs, and custom AI accelerators for training and inference.
Optimization techniques, including model compression, quantization, and pruning, improve performance and reduce computational overhead. AI is deployed across domains such as computer vision for image and video analysis, speech recognition for voice interfaces, natural language understanding for chatbots and automated translation, robotics for autonomous control, and scientific computing for simulations and drug discovery.
Advancements in federated learning, edge AI, and neuromorphic computing are driving AI’s scalability, adaptability, and real-time processing capabilities, enabling efficient and decentralized deployment in sectors such as healthcare, finance, manufacturing, and autonomous systems.
Checkout this playlist Introduction to AI (2024), which includes seven videos.
Click on the titles for the link.
Dates such as 2023.09.00 just means that it was published some time in September 2023.
By now everyone has heard about large language models (LLMs) even if they don’t fully understand that they are. This article tells us that “Training a model with hundreds of billions of parameters takes huge computational resources. To train its Gemini 1.0 Ultra model, for example, Google reportedly spent $191 million(opens a new tab). Large language models (LLMs) also require considerable computational power each time they answer a request, which makes them notorious energy hogs. A single query to ChatGPT consumes about 10 times(opens a new tab) as much energy as a single Google search, according to the Electric Power Research Institute”.
It goes on to argue that small language models can run on a laptop or cellphone. Of course ‘small’ still means a maximum of “around 10 billion parameters”. The idea is that it’s possible to ‘prune’ a LLM to keep only high-quality data, possibly for a more tightly defined topic space. It’s based upon the idea that “up to 90% of the parameters in a trained neural network could be removed without sacrificing efficiency”.
The BBC conducted a study evaluating the accuracy of AI chatbots, specifically ChatGPT, Google Gemini, Microsoft Copilot, and Perplexity, in summarizsing news content. The research revealed that over half of the AI-generated responses contained significant issues, including factual inaccuracies and distortions. Approximately 19% of the answers included incorrect statements, numbers, or dates, and 13% featured altered or nonexistent quotes attributed to the BBC. Notably, Google Gemini’s outputs were particularly concerning, with 46% flagged for accuracy problems. These findings underscore the challenges AI chatbots face in accurately conveying current affairs and highlight the need for collaboration between media organizations and AI developers to enhance information reliability.
The article highlighted fundamental limitations in large language models (LLMs), such as ChatGPT, particularly in performing compositional reasoning tasks. These tasks require combining solutions to subproblems to form a comprehensive answer, exemplified by logic puzzles like “Einstein’s riddle“. Research at the Allen Institute for AI found that LLMs trained solely to predict the next word in a sequence struggle with such tasks, often providing approximate or incorrect solutions. This suggests inherent limitations in transformer-based architectures, prompting discussions about exploring alternative approaches to overcome these challenges.
“Humanity’s Last Exam” (HLE), a benchmark developed by the Center for AI Safety (CAIS) and Scale AI to evaluate the capabilities of advanced AI models from organizations like OpenAI, Google, and Anthropic. The benchmark comprises over 3,000 multiple-choice and short-answer questions across more than 100 subjects, including complex topics such as Palmyrene script translation and hummingbird anatomy. These questions were sourced from nearly 1,000 subject matter experts worldwide, aiming to challenge AI models at the frontier of human knowledge.
In evaluations, leading AI models, including OpenAI’s GPT-4o and o1, Google’s Gemini 1.5 Pro, and Anthropic’s Claude Sonnet-3.5, scored below 10% on the HLE benchmark. This performance is notably lower than their results on other benchmarks like the Massive Multitask Language Understanding (MMLU) and Graduate-Level Google-Proof Q&A (GPQA), where these models have previously excelled.
The HLE benchmark is designed to be the final closed-ended academic assessment of its kind, with broad subject coverage. It aims to test AI models on problems that are at the frontier of human knowledge and reasoning. The benchmark includes questions that require both image and text comprehension, with approximately 10% of the questions being multimodal.
The development of HLE addresses the rapid advancement of AI models, which have begun to surpass existing evaluation benchmarks. By providing a more challenging and comprehensive assessment tool, HLE aims to better measure the current capabilities and limitations of AI systems.
This was an FT “opinion” that argued that “It’s not killer robots we should be worried about, but the automated plumbers of the information network”. It’s not the Big Robot Rebellion we should worry about, but the “digital bureaucrats”. Historically bureaucracy has been responsible for changing human societies in radical and unexpected ways, e.g. the invention of writing, the creation of ownership with individual property rights, the levying of taxes, the creation of paid armies and the establishment of large centralised states. Bureaucracy is an artificial environment, in which mastery of a narrow field is enough to exert enormous impact on the wider world, by manipulating the flow of information.
The author argued that in the coming years, millions of AI bureaucrats will increasingly make decisions about everything touching our lives, e.g. getting a loan, entry to university, getting a job, or being condemned or going free. AI could provide superior healthcare, education, justice and security, but what if things go wrong?
Algorithms that pursuit user engagement discovered that greed, hate and fear increase user engagement. Is this far-fetched? The author suggests not, and noted that Jean-Paul Marat shaped the course of the French Revolution by editing the influential newspaper L’Ami du Peuple. Eduard Bernstein shaped modern social democratic thinking by editing Der Sozialdemokrat. The most important position Vladimir Lenin held before becoming Soviet dictator was editor of Iskra. Benito Mussolini gained fame and influence as editor of the firebrand rightwing paper Il Popolo d’Italia. It is interesting that one of the first jobs in the world to be automated by AIs hasn’t been taxi drivers or textile workers, but news editors. The job that was once performed by Lenin and Mussolini can now be performed by AIs.
This is a pointer to a BBC article which was surprisingly interesting. It’s a topic I understand (at least superficially), but have no particular interest in. However the article covers all the basics and is easy to read. What did surprise me was the extent to which these AI companions are being adopted, and how (apparently) they manage to insert themselves into real peoples’ everyday lives.
You can see how developers could create chatbots that use love bombing tactics. I also agreed with the authors that advertising (explicit and subliminal) and product placements are inevitable. You can see AI companions as being an extension-evolution of the influencer, but will they exploit AI technology, or will they be replaced by personalised AI chatbot-influencers?
The article “OpenAI says it stopped multiple covert influence operations that abused its AI models” mentions generative AI being used to generate text and images at much higher volumes than before, and is being used to generate fake comments on social media posts. The culprits were Russia, China, Iran and Israel.
2024.05.14 AI is poised to drive 160% increase in data center power demand
In an article entitled “AI’s ‘insatiable’ electricity demand could slow its growth a lot, Arm exec says, “data centres powering AI chatbots already account for 2% of global electricity consumption“. It would appear that ChatGPT requires 15 times more energy than a traditional web search. A separate report estimated that energy consumption from hardware in data centres will more than double in the years ahead, according to Reuters, from 21 gigawatts in 2023 to more than 50 gigawatts in 2030.
The article also mentioned that there were already 9,000-11,000 cloud data centres across the globe, and for 2024 it was estimated that they would consume 46 terawatt-hours, three times more than 2023.
“Electricity grids creak as AI demands soar” is another article on how AI is pushing the demand for electricity.
Generative AI systems create content (answers) from scratch and use around 33 times more energy than machines running task-specific software. In 2022, data centres consumed 460 terawatt hours of electricity, and this will double over the next four years. Data centres could be using a total of 1,000 terawatts hours annually by 2026.
Interesting how the figures are inconsistent between the two articles.
What are the implications of generative Artificial Intelligence (AI) in creating synthetic moving images, particularly focusing on their potential use in online disinformation campaigns and the impact on historical footage archives? Deepfakes can make individuals appear to say or do things they never did, posing significant risks such as harassment, intimidation, extortion, misinformation campaigns, and undermining public trust.
AI-manipulated content, especially videos, will significantly influence the current information warfare landscape and affect visual historical records.
The article looks at the limitations of current artificial intelligence (AI) models, particularly large language models (LLMs), in achieving true artificial general intelligence (AGI). The author draws an analogy to “cargo cults“, the ritualistic practices that mimic the appearance of a desired outcome without understanding the underlying principles, to argue that while LLMs can replicate certain aspects of human intelligence, they lack the foundational ability to reason scientifically.
The article emphasizes that contemporary AI models are predominantly data-driven, relying on deep learning techniques applied to vast datasets. This approach enables them to perform tasks such as image recognition and natural language processing by identifying patterns within the data. However, these models do not possess the capacity for abstract reasoning, causal inference, or the formulation of universal principles that characterize human scientific thought. For instance, while a neural network trained on specific astronomical data might predict planetary positions, it would not derive the underlying laws of motion governing those positions.
The article underscored the significant financial investments required for generative AI, encompassing expenses related to specialized hardware, data storage, and ongoing maintenance. The three largest hyperscalers (Amazon, Microsoft, and Google) have reported large increases in datacenter CapEx investment from $78B in 2022 to $120B in 2023, a 54% increase.
From May 2022 to April 2023 (12 months), Nvidia’s datacenter group sold $15.5B of GPUs. During a May 24 conference call earlier this year, the firm doubled guidance for the next quarter, increasing quarterly datacenter GPU sales forecasts from $4B to $8B (to an annual rate of $32B).
The report highlighted the considerable energy demands of training large AI models, which can consume terawatt-hours of electricity, thereby raising concerns about sustainability and the strain on power grids. Estimating the annual power consumption of $32B of GPUs involves GPU prices, operational parameters, and associated server and datacenter power. Using A100 prices ($10k) and 300W average incremental load (GPU at 50% of TDP, server balance of 50%, and PUE of 1.0:3.2M GPUs → 960MW → 8.4 TWh/year.
To put this into perspective, 8.4 TWh/year was 54% of Google’s total datacenter power in 2020. Nvidia’s sales-rate increase alone corresponds to 27% of Google’s total 2020 fleet. A more complete comparison is difficult as several hyperscalers have stopped disclosing total power consumption due to growing public outcry. 8.4 TWh corresponds to 0.25% of the USA’s annual electric power consumption.
The article pointed out that the energy consumption associated with AI model training leads to substantial carbon-dioxide emissions, contributing to environmental degradation and climate change- Using 2021 U.S. average grid emissions, 8.4 TWh is 3.25 gigatons of carbon-dioxide, the equivalent of five billion U.S. cross-country flights. In high-penetration areas, cloud datacenters already exceed 20% of grid power. Their further growth is being limited by grid reliability risks.
The Birth of AI and the Cybernetics Era (1940s–1950s)
- Von Neumann’s Influence (1945):
- In First Draft of a Report on the EDVAC, John von Neumann laid the foundation for modern computer architecture.
- His work was influenced by cybernetics and early discussions on the equivalence of biological and mechanical intelligence.
- He described digital computer components using biological terms such as neurons, organs, and memory.
- Edmund Berkeley’s Giant Brains (1949):
- Berkeley popularized the idea of computers as “thinking machines” and speculated on their future role in machine translation, speech recognition, and psychological therapy.
- His work framed computers as being fundamentally capable of human-like thought, which was later challenged.
The Dartmouth Conference and the Birth of AI (1956)
-
John McCarthy, Marvin Minsky, Claude Shannon, and others organized the Dartmouth Summer Research Project on Artificial Intelligence.
- This event formally introduced the term Artificial Intelligence (AI).
- Defined AI as making machines capable of human-like reasoning, abstraction, and self-improvement.
-
Herbert Simon and Allen Newell’s “Thinking Machine” (1955–1956):
- They developed Logic Theorist, the first AI program capable of proving mathematical theorems.
- It introduced heuristic search and problem-solving approaches that influenced later AI.
-
Alan Turing’s Imitation Game (1950):
- Proposed the Turing Test as a practical measure of machine intelligence.
- Turing predicted AI would be widely accepted within 50 years.
AI and the Formation of Computer Science (1950s–1960s)
-
AI was instrumental in the creation of computer science as an academic discipline.
- Researchers like McCarthy, Minsky, Newell, and Simon founded AI labs at top universities:
- MIT AI Lab (1958)
- Stanford AI Lab (1966)
- Carnegie Mellon University AI Research Program (1965)
- AI was one of the most prestigious subfields in the emerging field of computer science.
- Researchers like McCarthy, Minsky, Newell, and Simon founded AI labs at top universities:
-
The Turing Award and AI Recognition (1966–1976):
- The first decade of Turing Awards honored AI pioneers:
- Marvin Minsky
- John McCarthy
- Allen Newell
- Herbert Simon
- This signified AI’s dominance in early computer science research.
- The first decade of Turing Awards honored AI pioneers:
Symbolic AI vs. Connectionism (1950s–1980s)
-
Rise of Symbolic AI:
- AI research in the 1960s and 1970s focused on symbolic reasoning and logic-based AI.
- Marvin Minsky’s “frame theory” became influential.
- Neural networks (connectionism) were sidelined after Minsky and Papert’s Perceptrons (1969) criticized their limitations.
-
AI Winter and the Decline of Symbolic AI (1970s–1980s):
- Funding cuts hit AI research due to failures in machine translation and robotics.
- Expert systems and knowledge-based AI emerged as a more practical alternative.
The Legacy of Early AI
-
Key Contributions of AI Research Beyond AI:
- John McCarthy invented:
- Garbage collection in programming.
- LISP, which became the primary AI programming language.
- Time-sharing computing, which evolved into modern cloud computing.
- John McCarthy invented:
-
AI’s Role in Advancing Computer Science Infrastructure:
- Many core computing concepts (interactive computing, heuristics, knowledge representation) originated in AI research.
- AI’s focus on building tangible, running systems contributed to major advancements in programming languages, optimization, and human-computer interaction.
2023.05.01 AlphaFold Spreads through Protein Science
AlphaFold, developed by DeepMind, has significantly impacted protein science by providing accurate predictions of protein structures from amino acid sequences.
AlphaFold 2 (2020) utilized deep learning techniques to predict protein structures with remarkable accuracy, often comparable to experimental methods. It modeled the physical and geometric constraints of protein folding, enabling the prediction of complex structures.
AlphaFold 3 (2024) extended its capabilities to predict interactions between proteins and other biomolecules, including DNA and RNA. It introduced the “Pairformer” architecture, which refined initial predictions through a diffusion model, enhancing the accuracy of complex formation predictions.
AlphaFold’s open-source code has been integrated into various protein-structure tools and workflows, becoming central to computational biology research. Its predictions have accelerated studies in areas such as enzyme mechanisms, protein-protein interactions, and drug target identification.
In recognition of this groundbreaking work, Demis Hassabis and John Jumper were awarded the 2024 Nobel Prize in Chemistry for their contributions to protein structure prediction.
Large language models (LLMs) find it difficult to understanding negation, a fundamental aspect of human language. LLMs are built upon neural network architectures that process language by predicting subsequent words in a sequence. These models are trained on vast datasets, enabling them to generate coherent and contextually relevant text. However, their training primarily focuses on the presence of data, leading to difficulties in comprehending the absence or negation of information.
Negation involves understanding the absence or opposite of a concept, which requires a grasp of context and the ability to infer implicit meanings. LLMs often struggle with sentences containing negations, leading to misinterpretations or incorrect responses. For instance, when processing a statement like “A bird cannot fly,” a model might fail to recognize the negation and incorrectly associate all birds with the ability to fly.
One problem is that LLMs encode language into mathematical representations, capturing relationships between words based on their co-occurrence in the training data. Negations, which denote the absence of a characteristic, are less frequently represented in the data, leading to weaker associations. The core objective of LLMs is to predict the next word in a sequence. This predictive mechanism emphasizes the presence of features and patterns, making it challenging for models to account for the absence or negation of information.
AI-generated art primarily utilizes machine learning models, notably Generative Adversarial Networks (GANs), to create images that can closely resemble human-made art. GANs consist of two neural networks, a generator and a discriminator, that work in tandem. The generator produces images from random noise, aiming to mimic the characteristics of real photographs. The discriminator evaluates the generated images against real images, providing feedback to the generator to improve its outputs. Through iterative training on extensive datasets of photographs and artworks, the generator learns to produce increasingly realistic images. This process has led to the creation of AI-generated images that are often indistinguishable from those captured or crafted by human artists.
The emergence of AI-generated art has sparked significant debate within the art community about authenticity and intellectual property. One problem is AI in art has outpaced existing legal frameworks, leading to calls for updated regulations to address issues of authorship, copyright, and the ethical use of AI in creative fields.
AI systems, especially advanced chess engines like AlphaZero developed by DeepMind, have revolutionized chess by introducing innovative strategies that challenge traditional principles. AlphaZero, for instance, employs deep neural networks and reinforcement learning to evaluate positions and make decisions, differing from earlier engines that relied heavily on brute-force calculations. This approach has led to unconventional tactics, such as sacrificing pieces to gain positional advantages, and using the king as an active piece earlier in the game.
These strategies have been adopted by top players, including former World Champion Magnus Carlsen, who have integrated AI-influenced tactics into their playbooks.
IBM has been a pioneer in the intersection of AI and chess. In the late 20th century, IBM developed Deep Blue, a chess-playing supercomputer that made history by defeating reigning World Chess Champion Garry Kasparov in a six-game match in 1997. This event marked the first time a computer defeated a world champion under standard chess tournament time controls. Deep Blue’s architecture relied on a combination of specialized hardware and software, enabling it to evaluate approximately 200 million positions per second. While it primarily used brute-force computation, it also incorporated strategic heuristics to assess positions, laying foundational work for future AI developments in chess.
The Turing Test and Early Machine Intelligence (1950s)
- Alan Turing’s “Imitation Game” (1950)
-
- Introduced the Turing Test, a method for assessing whether a machine can exhibit human-like intelligence.
- Sparked discussions about machine consciousness and whether computers could truly think and feel.
- This idea influenced later AI developments and philosophical debates about artificial intelligence.
ELIZA and the First Chatbots (1960s)
- Joseph Weizenbaum’s ELIZA (1964)
-
- One of the first AI programs to simulate human conversation.
- Used pattern-matching to mimic a Rogerian psychotherapist.
- Many users believed ELIZA was genuinely sentient, despite its simplistic rule-based responses.
- Demonstrated how easily people could be deceived by human-like interactions with machines.
The Rise of AI Language Models and Sentience Debates (2020s)
-
Blake Lemoine and Google’s LaMDA (2022)
- Lemoine, a former Google engineer, claimed LaMDA (a conversational AI model) was sentient.
- Led to public discussions and backlash from AI researchers, including John Etchemendy and Stuart Shieber.
- Highlighted the growing sophistication of AI-generated language and the ethical challenges surrounding AI sentience.
-
“Stochastic Parrots” Debate (2021)
- A research paper by Timnit Gebru and colleagues criticized large AI language models, calling them “stochastic parrots“.
- Argued that AI systems generate human-like text without understanding meaning.
- Raised concerns about bias, misinformation, and ethical risks in AI-driven language models.
The Expansion of AI Language Models
-
OpenAI’s GPT-3 (2020)
- Demonstrated remarkable fluency in generating text, answering research questions, and even writing fiction.
- Despite its abilities, GPT-3 still produces inaccuracies and nonsensical text, highlighting the limitations of AI understanding.
-
Advancements in AI Training Techniques
- Use of word vectors to mathematically represent words and their relationships.
- Recognizing Textual Entailment (RTE) to classify relationships between sentences.
- Companies like Google, DeepMind, Meta, Microsoft, and Amazon continue pushing AI language capabilities.
Ethical and Future Challenges
- Concerns about AI Safety and Bias
- Research has shown that large AI models can generate racist, sexist, and toxic language.
- Ethical AI organisations like DAIR (Distributed AI Research) and OpenAI are exploring solutions.
- The Future of AI Linguistics
- AI-driven virtual assistants, chatbots, and robots are improving human-computer interaction.
- Future applications may transform education, customer service, and human-AI collaboration.
- Researchers emphasize that true sentience and reasoning in AI remain distant goals.
ChatGPT is based on GPT-3.5, a variant of OpenAI’s Generative Pre-trained Transformer (GPT) models, optimized for conversational interactions. It follows a transformer-based architecture with deep learning techniques for natural language understanding and generation.
The model is pre-trained on large-scale datasets comprising diverse internet text. Then human AI trainers provide conversations in which they play both user and assistant roles, and then rank model-generated responses. Proximal Policy Optimisation (PPO) is used to improve response quality iteratively.
The objective is to generate human-like text responses in a conversational manner. However, it can generate incorrect or nonsensical answers, and may produce biased responses due to underlying training data.
This is about a 100-year effort to study and anticipate how the effects of artificial intelligence will ripple through every aspect of how people work, live and play. The 2016 report noted that Artificial Intelligence (AI) is a science and a set of computational technologies that were already impressive, but were highly tailored to particular tasks.
Contrary to the more fantastic predictions for AI in the popular press, the report concluded that there was no cause for concern that AI was an imminent threat to humankind. No machines with self-sustaining long-term goals and intent had been developed, nor were they likely to be developed in the near future. At the same time, the report also concluded that many AI developments would spur disruptions in human labour markets, creating new challenges for the economy and society more broadly.
In the 2021 report it was noted that AI had made remarkable progress in the period 2016-2021 and was already having real-world impact on people, institutions and culture. The ability of computer programs to perform sophisticated language- and image-processing tasks, core problems that have driven the field since its birth in the 1950s, had advanced significantly.
The field’s successes had led to an inflection point, meaning that it was urgent to think seriously about the downsides and risks that the broad application of AI was revealing. The increasing capacity to automate decisions at scale was seen as a double-edged sword. Both intentional deepfakes or simply unaccountable algorithms making mission-critical recommendations can result in people being misled, discriminated against, and even physically harmed. Algorithms trained on historical data are disposed to reinforce and even exacerbate existing biases and inequalities.
Governments were expected to play a critical role in shaping the development and application of AI, but they were still behind the curve, and sustained investment of time and resources was needed to meet the challenges posed by rapidly evolving technology. Governments needed to look ahead to ensure the creation of informed communities, and they also needed to include AI concepts and implications into school education.
This article becomes quite technical, quite quickly, but the important point for me was the question about the trustworthiness of the input data.
Machine learning, a subfield of artificial intelligence, has advanced radically over the past 10 years, and machine learning algorithms now achieve human-level performance or better on a number of tasks, including face recognition, optical character recognition, object recognition, and playing the game Go. Machine learning is already used for many highly important applications and will be used in even more of even greater importance in the near future. Search algorithms, automated financial trading algorithms, data analytics, autonomous vehicles, and malware detection are all critically dependent on the underlying machine learning algorithms that interpret their respective domain inputs to provide intelligent outputs that facilitate the decision-making process of users or automated systems.
As machine learning is used in more contexts where malicious adversaries have an incentive to interfere with the operation of a given machine learning system, it is increasingly important to provide protections, or ‘robustness guarantees’, against adversarial manipulation.
Most modern machine learning can essentially be understood as applied function approximation. That is when there is some mapping from an input x to an output y that is difficult for a programmer to describe through explicit code, a machine learning algorithm can learn an approximation of the mapping by analysing a dataset containing several examples of inputs and their corresponding outputs.
To get an idea of what this means we can look at Google’s image-classification system which has been trained with millions of labeled images. It can classify images as cats, dogs, airplanes, boats, or more complex concepts on par or improving on human accuracy. Increases in the size of machine learning models and their accuracy is the result of recent advancements in machine learning algorithms, particularly to advance deep learning. The accuracy of the system varies greatly with the choice of the dataset used to train it.
Machine learning has traditionally been developed following the assumption that the environment is benign during both training and evaluation of the model. Specifically, the inputs x are usually assumed to all be drawn independently from the same probability distribution at both training and test time. This means that while test inputs x are new and previously unseen during the training process, they at least have the same statistical properties as the inputs used for training. Such assumptions have been useful for designing effective machine learning algorithms but implicitly rule out the possibility that an adversary could alter the distribution at either training time or test time.
Herein, is the topic of this article. What happens when an adversary chooses a distribution at test time that is designed to be exceptionally difficult for the model to process accurately. For example, an adversary might modify an image (slightly) to cause it to be recognised incorrectly or alter the code of an executable file to enable it to bypass a malware detector.
The study of adversarial examples is in its infancy, and the article is an overview of the foundations and advancements of this research field and some predictions of where it might lead.
I’ve added this article from 2016 as context to the AI100 study mentioned above.
At the time the author mentioned the ‘great excitement’ felt after the recent success of neural networks in applications such as speech recognition, vision and autonomous navigation.
Some people were suggesting that the automation of these tasks could soon reach human level intelligence.
The author asked if a system that does not require any explicit modelling or sophisticated reasoning, could be sufficient for reproducing human level intelligence.
The author described two distinct approaches for tackling problems that have been of interest to AI, the first approach model-based and the second function-based. Consider the object recognition and localisation task involving a dog wearing a hat.
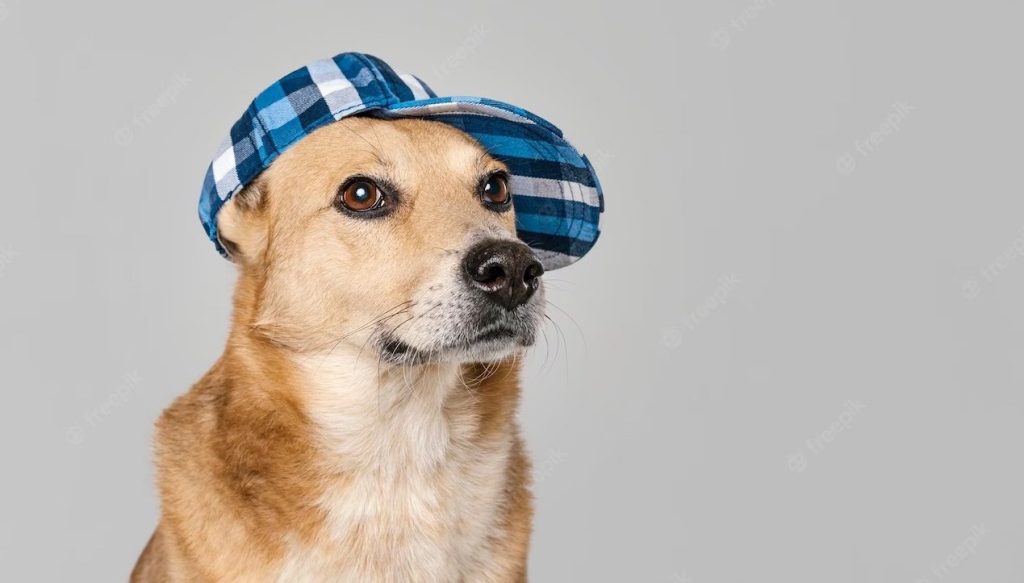
To solve this recognition task, the model-based approach requires one to represent knowledge about dogs and hats, among other things, and involves reasoning with such knowledge. The main tools of this approach today are logic and probability (mathematical modeling more generally) and it can be thought of as the represent-and-reason approach originally envisioned by the founders of AI. It is also the approach normally expected, at some level, by the informed member of the scientific community.
The function-based approach on the other hand formulates this as a function-fitting problem, with the function inputs coming directly from the image pixels and its outputs corresponding to the high-level recognitions we are seeking (the function must have a form that can be evaluated efficiently). The main tool of this approach today is the neural network.
The main difference here is that functions are now employed that have multiple inputs and outputs. The structure of these functions can be arbitrarily complex, and the problems are associated with cognition, as opposed to, say, estimating the relationship between two variables in a engineered system.
The main observation in AI was that the function-based approach was quite effective at certain AI tasks, more so than the model-based approach. This surprised not only mainstream AI researchers, who mainly practiced the model-based approach, but also machine learning researchers who practiced various approaches, of which the function-based approach was just one. This has had many implications, some positive and some giving grounds for concern.
On the positive side is the increasing number of tasks and applications that are now within reach, using a tool that can be very familiar to someone with only a broad engineering background, particularly, those people who are accustomed to estimating functions and using them to make predictions. What is of concern, however, is the current imbalance between exploiting, enjoying and cheering this tool on the one hand, and thinking about it on the other hand. This thinking is not only important for realizing the full potential of this tool, but also for scientifically characterizing and understanding its potential reach. The lack of such characterisation, or at least the attempt to seek it aggressively enough, was seen as being responsible for current misconceptions about AI progress and where that may lead us in the future.
The author argued that what we are actually seeing is ‘automation‘ more than ‘AI’, as the latter is just a technology that happens to improve and speed up automation. As such, to address these concerns, the focus should be shifted towards policy and regulatory considerations for dealing with the new level of automation that our society is embarking on, instead of fearing AI.
The author also noted the change in how we measure success.
Consider machine translation for example, which received significant attention in the early days of AI. The represent-and-reason approach is considered to have failed on this task, with machine learning approaches being the state of the art now (going beyond function-based approaches). In the early days of AI, success was measured by how far a system accuracy was from 100%, with intelligence being a main driving application (a failure to translate correctly can potentially lead to a political crisis). The tests that translation systems were subjected to compared their performance to human translation abilities (e.g., translate a sentence from English to Russian then back to English). Today, the almost sole application of machine translation is to web content. Here, success is effectively measured in terms of how far a system accuracy is from 0%. If I am looking at a page written in French, a language that I don’t speak, I am happy with any translation that gives me a sense of what the page is saying. In fact, the machine translation community rightfully calls this ‘gist translation’. It can work impressively well on prototypical sentences that appear often in the data, but can fail badly on text drawn from a novel. This is still very valuable, yet it corresponds to a task that is significantly different from what was tackled by early AI researchers. Current translation systems will fail miserably if subjected to the tests adopted by early translation researchers. Moreover, these systems will not be suitable, or considered successful, if integrated within a robot that is meant to emulate human behavior or abilities.
The authors thoughts as expressed in the title of his article, is that it’s wrong to attributing human level intelligence to the tasks currently conquered by neural networks. The tasks barely rise to the level of abilities possessed by many animals. Eagles and snakes have vision systems that surpass what we can build today. Cats have navigation abilities that are far more superior to any of the ones possessed by existing, automatous navigation systems including self driving cars. Dogs can recognize and react to human speech and African grey parrots can generate sounds that mimic human speech to impressive levels. Yet none of these animals posses the cognitive abilities and intelligence typically attributed to humans.
If you have got this far, check out the article to see what the author thinks is the next step on the long road to understanding human cognitive functions.